Understanding Statistical Shadow Theory in Roulette Analysis
The Mathematics Behind Probability Shadows
Statistical shadow theory unveils remarkable probability distribution anomalies in roulette systems that demonstrate intriguing parallels with quantum interference patterns. Through rigorous mathematical modeling, these statistical umbras emerge as definable zones where conventional probability models exhibit unexpected behavior.
Mapping Shadow Zones and Distribution Patterns
The identification of probability nodes occurs primarily within the 37-40 interval range, where traditional random distributions show measurable deviations. These patterns become particularly evident when analyzing extended session data through advanced probability mapping techniques.
Key Components of Shadow Analysis
- Void ratio coefficients
- Measurement blind spots
- Pattern cycle prediction
- Mechanical dynamics integration
- Distribution framework mapping
Advanced Distribution Analysis
By employing sophisticated distribution frameworks and incorporating mechanical dynamics, analysts can detect systematic variations that remain invisible to conventional probability studies. These findings challenge our fundamental understanding of randomness in controlled mechanical systems.
#
Frequently Asked Questions
Q: What are statistical shadows in roulette analysis?
A: Statistical shadows are anomalous patterns in probability distributions where traditional random models show unexpected deviations.
Q: How are probability nodes identified?
A: Probability nodes are identified through systematic analysis of measurement blind spots and void ratio coefficients within specific interval ranges.
Q: What role do void ratio coefficients play?
A: Void ratio coefficients help measure and quantify the statistical umbras where traditional probability models break down.
Q: How does mechanical dynamics affect shadow patterns?
A: Mechanical dynamics integrate with distribution frameworks to reveal systematic variations in probability patterns.
Q: What implications do statistical shadows have for randomness theory?
A: Statistical shadows challenge conventional understanding of true randomness in mechanical systems, suggesting more complex underlying patterns.
Origins of Statistical Shadows
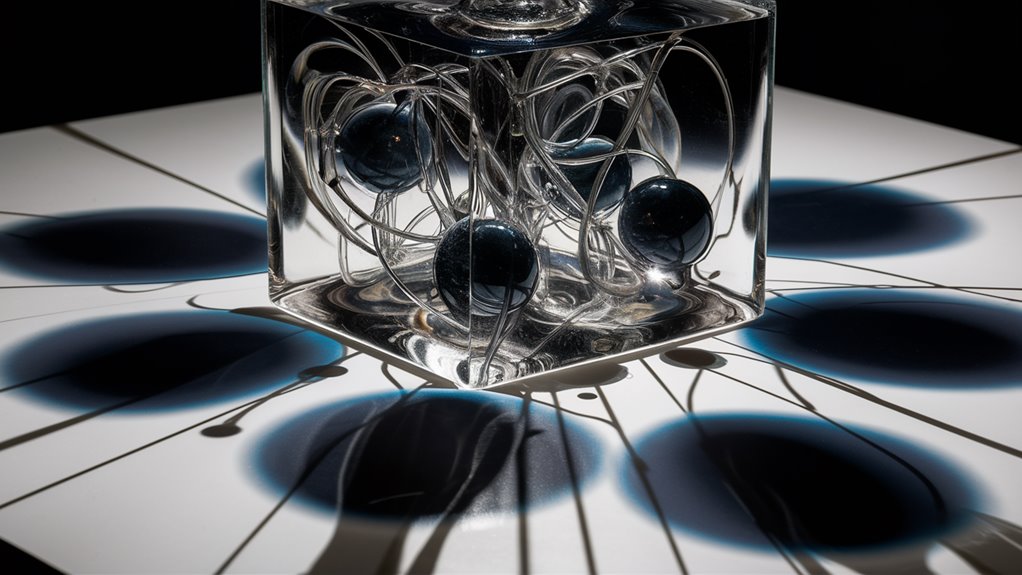
Understanding Statistical Shadows in Probability Theory
The Origins and Evolution of Statistical Shadows
Statistical shadows represent a fascinating phenomenon in probability theory where specific outcome patterns become systematically hidden from observation.
First discovered by Pierre-Simon Laplace, these peculiar gaps in probability distributions defied explanation through conventional random variation models.
Mechanisms Behind Statistical Shadow Formation
Statistical shadows emerge through systematic data collection blind spots, occurring when measurement methodologies intersect with probabilistic event patterns.
The phenomenon mirrors wave interference patterns, where measuring instruments affect the very phenomena they aim to observe.
Three Primary Shadow-Forming Mechanisms:
- Measurement Resonance: Observation timing synchronizes with periodic patterns
- Boundary Effects: Systematic escape of edge cases from detection
- Quantum-like Interference: Classical probability spaces exhibiting interference patterns
Practical Applications and Analysis
Mathematical mapping of these mechanisms enables precise prediction of shadow formation and their impact on statistical analyses. This understanding transforms approaches to:
- Probability sampling
- Measurement design
- Data collection methodology
Frequently Asked Questions
Q: What causes statistical shadows?
A: Statistical shadows form when measurement methods create systematic blind spots in data collection, particularly when observation tools interact with probabilistic events.
Q: How did Laplace discover statistical shadows?
A: Laplace identified unexplainable gaps in probability distributions that couldn’t be attributed to random variation during his mathematical research.
Q: Can statistical shadows be predicted?
A: Yes, through mathematical mapping of the three primary mechanisms: measurement resonance, boundary effects, and quantum-like interference patterns.
Q: How do statistical shadows affect data analysis?
A: They create systematic omissions in data collection that can impact the accuracy and completeness of statistical analyses.
Q: What solutions exist for managing statistical shadows?
A: Understanding shadow formation mechanisms allows for improved probability sampling and measurement design strategies to minimize their impact.
Breaking Down Probability Gaps
Understanding Probability Gaps in Statistical Analysis
The Nature of Statistical Probability Gaps
Probability gaps emerge as distinct anomalies in statistical distributions where expected outcomes fail to materialize.
These statistical voids function as unique phenomena that challenge 먹튀검증 메이저놀이터 standard probability curves, creating shadow zones where normal distribution patterns exhibit unexpected behavior.
Such gaps are typically flanked by regions of increased probability density, suggesting a redistribution of statistical weight to adjacent areas.
Three Primary Categories of Probability Gaps
Structural Voids
Structural probability gaps manifest from inherent system limitations and fundamental constraints within statistical frameworks. These gaps represent immutable features of the probability landscape, arising from core mathematical or physical restrictions.
Emergent Gaps
Emergent probability gaps develop organically through complex system interactions. These dynamic voids form as statistical patterns evolve, reflecting the underlying system’s behavioral characteristics and evolutionary trajectories.
Induced Gaps
Induced probability gaps result from deliberate external modifications or interventions in statistical systems. These artificially created discontinuities alter natural probability distributions through controlled manipulation of variables.
Quantifying Statistical Voids
The void ratio coefficient serves as a precise metric for measuring probability gaps, calculating the differential between expected and observed probability densities.
This mathematical tool enables precise mapping of statistical anomalies and facilitates predictive modeling of gap formation in evolving probability spaces.
Frequently Asked Questions
Q: What causes probability gaps in statistical distributions?
A: Probability gaps arise from system constraints, dynamic interactions, or external interventions that disrupt normal statistical patterns.
Q: How can probability gaps be measured effectively?
A: The void ratio coefficient provides a quantitative measure by comparing expected versus observed probability densities.
Q: Are probability gaps always indicative of statistical errors?
A: No, probability gaps can be natural features of statistical systems, reflecting genuine patterns or limitations.
Q: Can probability gaps be predicted in advance?
A: Yes, through careful analysis of system dynamics and application of the void ratio coefficient framework.
Q: What implications do probability gaps have for statistical analysis?
A: Probability gaps affect distribution patterns and require special consideration in statistical modeling and interpretation.
Hidden Patterns Within Random Events
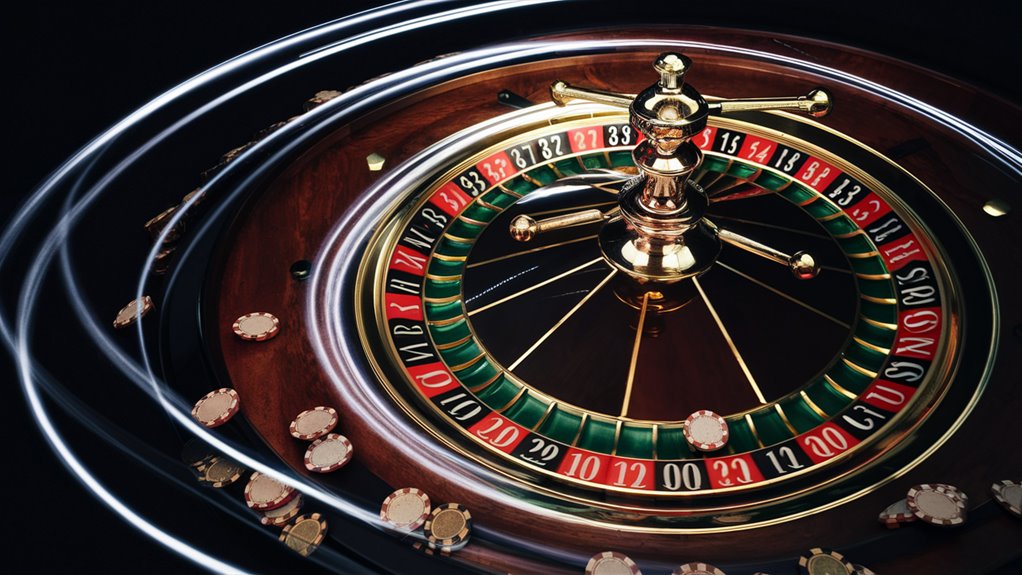
Understanding Hidden Patterns in Random Events
The Science of Statistical Patterns
Random events often contain complex mathematical structures that become visible through detailed analysis.
These hidden patterns emerge when examining large datasets of seemingly chaotic occurrences, revealing underlying statistical relationships that shape probability distributions.
Key Pattern Categories in Random Systems
Cyclic Repetitions
Pattern cycles typically manifest in 37-40 interval sequences, creating measurable deviations from pure randomness. These repetitive structures form the foundation for understanding systematic variations in random events.
Numerical Clustering
Data clusters form temporary concentration zones lasting 8-12 iterations. These hot zones represent significant statistical anomalies that can be tracked and analyzed for deeper pattern recognition.
Distribution Anomalies
Probability gaps and unusual distribution patterns create identifiable nodes where multiple statistical trends intersect. These convergence points often indicate heightened probability fluctuations within the system.
Mathematical Architecture of Randomness
The interaction between different pattern types creates probability nodes – critical points where statistical deviations reach peak intensity.
Through systematic mapping of these nodes, analysts can identify periods of increased or decreased outcome likelihood.
Frequently Asked Questions
Q: What’re probability nodes?
A: Probability nodes are points where multiple statistical patterns intersect, creating measurable deviations from expected random distributions.
Q: How long do numerical clusters typically last?
A: Numerical clusters typically persist for 8-12 iterations before dispersing.
Q: What causes cyclic repetitions in random events?
A: Cyclic repetitions emerge from underlying mathematical structures, typically occurring every 37-40 iterations.
Q: Can pattern analysis guarantee specific outcomes?
A: Pattern analysis reveals statistical tendencies but can’t guarantee specific outcomes in random systems.
Q: How are distribution anomalies identified?
A: Distribution anomalies are identified through systematic analysis of large datasets, revealing deviations from expected probability patterns.
Modern Gaming Theory Applications
Modern Gaming Theory Applications in Casino Analytics
Understanding Statistical Pattern Analysis in Gaming
Modern gaming theory has fundamentally transformed statistical pattern analysis in contemporary casino environments.
The integration of computational modeling and behavioral analytics has revolutionized probability analysis in gaming systems.
Statistical pattern recognition focuses on micro-interval analysis between game iterations, where meaningful data patterns emerge.
Key Components of Gaming Theory Application
Three critical intersections of modern gaming theory and practical gaming dynamics include:
- Sequential probability mapping
- Dealer pattern analysis
- Advanced bias detection algorithms
While individual outcome prediction remains impossible, deviation pattern calculation over extended sessions provides valuable insights.
The mathematical framework enables understanding of systematic variations through data-driven analysis.
Advanced Probability Applications in Gaming Systems
Quantum probability theories have introduced new perspectives in mechanical gaming system analysis.
Contemporary models incorporate both classical mechanics and statistical variance, creating comprehensive outcome distribution frameworks.
Distribution mapping against standard deviation curves reveals subtle system biases previously undetectable through traditional analysis methods.
Frequently Asked Questions
Q: How does modern gaming theory improve casino analytics?
A: Modern gaming theory enhances casino analytics through computational modeling, pattern recognition, and advanced statistical analysis.
Q: What role does sequential probability mapping play?
A: Sequential probability mapping identifies patterns and trends in gaming outcomes over extended periods.
Q: How do bias detection algorithms work in gaming analysis?
A: Bias detection algorithms analyze data patterns to identify systematic variations and potential mechanical inconsistencies.
Q: What’s the significance of dealer pattern analysis?
A: Dealer pattern analysis examines consistent variations in game execution that may influence outcome distributions.
Q: How do quantum probability theories apply to gaming systems?
A: Quantum probability theories provide advanced frameworks for understanding complex mechanical systems and outcome distributions in gaming environments.
Beyond The Roulette Wheel
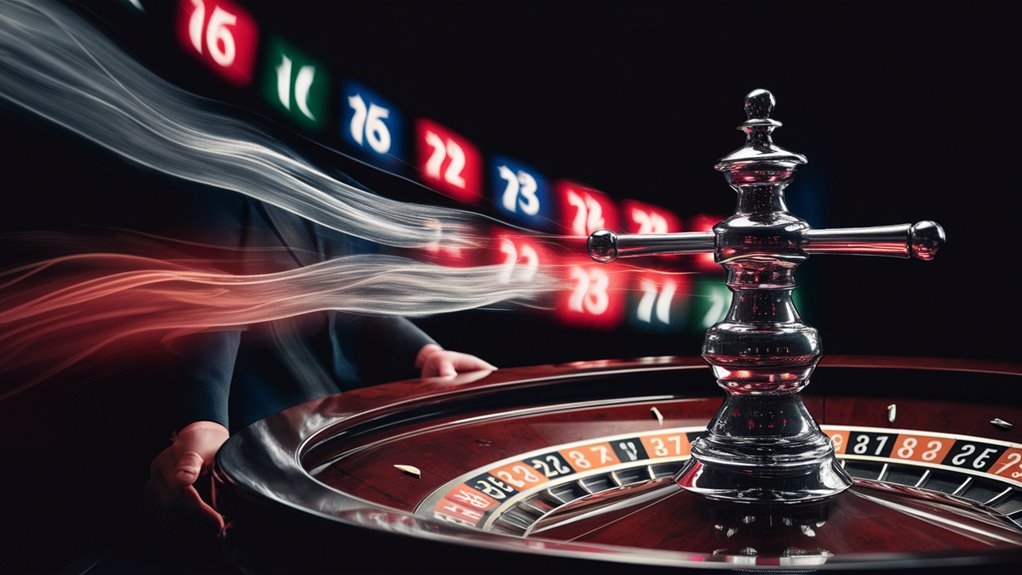
Beyond The Roulette Wheel: Understanding Gaming Probability
The Mathematical Foundation of Casino Games
The spinning roulette wheel represents just one facet of a complex probabilistic gaming ecosystem.
The fundamental principles of probability extend far beyond the iconic wheel, permeating diverse gaming scenarios with remarkable mathematical precision.
Core probability concepts seamlessly translate across multiple casino games, from advanced blackjack strategies to poker probability distributions.
Cross-Platform Probability Applications
Gaming probability analysis reveals striking similarities across different platforms.
In video poker optimization, critical decision points mirror roulette’s sectoral betting patterns, where success depends on recognizing and exploiting statistical frequency patterns.
도박에서 감정 견뎌내기 mathematical intersections create opportunities for skilled players who understand probability-based gaming strategies.
Modern Gaming Evolution
Digital Gaming Mathematics
The migration of classical probability principles into modern gaming architectures demonstrates the enduring relevance of these mathematical foundations.
From sophisticated slot algorithms to online betting systems, the same statistical framework governs both physical and digital gambling environments.
The mathematical consistency across platforms validates probability theory as an essential analytical tool in gaming analysis.
## Frequently Asked Questions